Key Points:
- Researchers used AI to screen through over 800,000 molecules to enrich for structurally diverse compounds with senolytic activity.
- Three candidates were more specific at killing senscent cells than a well known senolytic drug ABT-737.
- One candidate compound, BRD-K56819078, significantly decreased senescent cell burden in aged mice.
Researchers from the Massachusetts Institute of Technology (MIT) and Harvard University used artificial intelligence (AI) to sort through hundreds of thousands of molecules in search of anti-aging drugs. Using data from the screening of 2,352 compounds for senolytic activity (the ability to kill cells that no longer replicate and divide often due to aging), the researchers were able to train a neural network to predict senolytic activity for over 800,000 molecules. This strategy uncovered chemical entities with higher predicted senolytic activity compared to currently available senotherapeutics. Specifically, the compound BRD-K56819078 reduced the number of senescent cells in older mice. These results highlight the potential of using AI to find senotherapeutics. This research was published in the journal Nature Aging.
The Concurrent Growth of Senolytic Research and AI
There has been a lot of focus on finding new senolytics because of their potential to promote healthy aging. Dasatinib, quercetin, fisetin, and ABT-263 were the first senolytics to be developed in the middle of the 2010s. These drugs were the result of targeted bioinformatics approaches that investigated the pathways that shield senescent cells from programmed cell death, keeping them in a “zombie”-like state. High-throughput screens and in-depth mechanistic studies have led to the discovery of senolytics like heat shock protein (HSP)-90 inhibitors, cardiac glycosides, and bromodomain and extra-terminal domain (BET) family protein inhibitors.
However, many of the currently available senolytics have undesirable side effects or limited clinical utility. Senolytics like fisetin and ABT-737, for example, have poor bioavailability. Patients in phase II studies of ABT-263 as a lung cancer treatment frequently had side effects such as having very few platelets (called thrombocytopenia) or white blood cells (called neutropenia). To further the development of senolytics as a class of therapeutics, it is necessary to identify novel senolytic compounds.
The development of senolytics has coincided with the widespread application of AI methods to speed up the drug discovery process. Different machine learning models have used training data from biological screens or public databases, as well as architectures like neural networks, to predict compound activities and drug properties, find molecular binding targets and age-related biomarkers, and design molecules that meet certain biological activity and physicochemical property criteria. After being discovered using machine learning techniques to treat conditions as diverse as bacterial infection and tissue scarring (fibrosis), these chemical compounds have room for further development, testing, and application in other therapeutic areas, including senolytics.
Discovering Small-Molecule Senolytics with AI
This prompted researchers in the lab of American bioengineer James J. Collins to suggest that AI could be taught to predict senolytic activity solely on the basis of a compound’s chemical structure. They reasoned that, as was demonstrated with antibiotics, AI could help high-throughput screening efforts by identifying senolytic compounds from extensive chemical spaces.
Lead authors Felix Wong and Satotaka Omori used the results of a screen for senolytic activity of 2,352 compounds to train a graph neural network platform, which was then used to predict senolytic activity in a chemical space containing 804,959 compounds. As a result of incorporating this strategy into high-throughput screens for compounds with senolytic activity, the working hit rate (positive predictive value) was increased by a factor of six. It is expected that increasing the number of active compounds used in model training will further improve prediction accuracy.
The researchers then honed in on three senolytic candidates to study in detail: BRDK20733377, BRD-K56819078, and BRD-K44839765. The results show that they are selective against senescent cells in models of therapy-induced and replicative senescence, with selectivity similar to that of ABT-737. Importantly, measurements of cellular viability over time show that all three compounds could selectively kill senescent cells without stopping the growth of untreated, healthy cells at certain concentrations, unlike ABT-737.
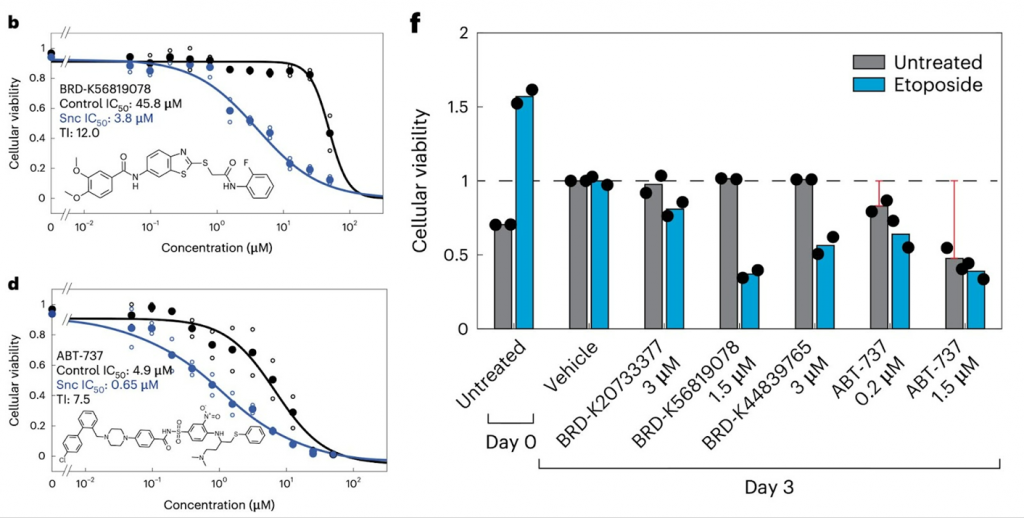
Furthermore, Collins and colleagues found that the identified compounds have encouraging safety profiles in initial toxicity tests and tested one compound, BRD-K56819078, in aged mice. BRD-K56819078 was extremely safe; all treated mice tolerated it well, and there were no obvious side effects in the form of toxicity, abnormal behavior, or abnormal weight loss. The kidneys of aged mice treated with BRD-K56819078 over two weeks revealed significant decreases in the areas of senescent positive cells in the kidney, with average decreases of ~20% relative to untreated mice.
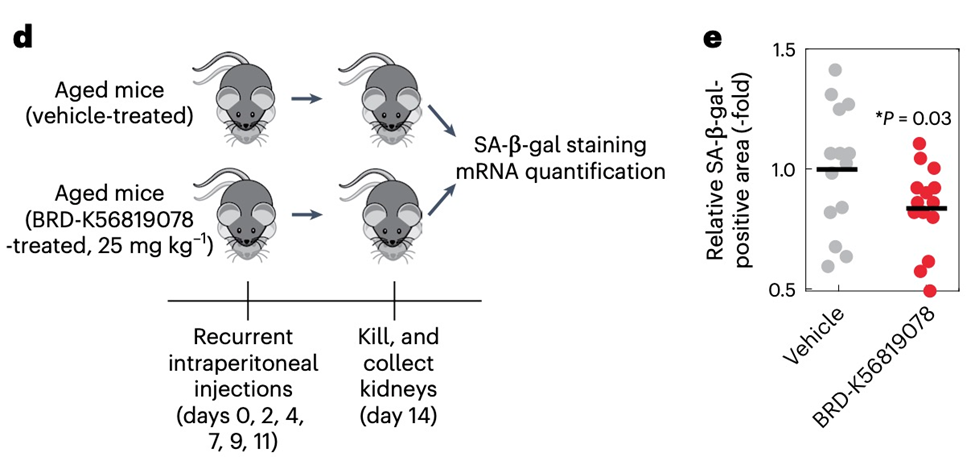
Limitations of AI in Drug Discovery
Taken together, these findings demonstrate that combining experiments with computation and deep learning can enable one to efficiently discover senolytic compounds and rapidly elucidate their potential mechanisms of action. It is important to highlight that the purpose of this approach is to facilitate lead generation. And while this AI approach is promising, it also has limitations. The training data used, which was crucial to this study, is what restricts machine learning approaches. Even though the screen of 2,352 compounds and activity criteria found enough active compounds to train models with predictive power, more structural diversity in the training set will help models figure out more accurately the chemical substructures that give senolytic activity. Similarly, this approach relies on training data generated from drug screens to make predictions of senolytic activity.
Nevertheless, these findings underscore the promise of leveraging deep learning to discover senotherapeutics. Additionally, there are some companies emerging in this space. For example, clinical-stage end-to-end generative artificial intelligence (AI)-driven drug discovery company Insilico Medicine has focused on the intersection of aging and disease since its inception, and Zhavoronkov is a prominent figure in the longevity space. Therefore, it is not difficult to foresee AI as an essential component for future life and health science research and for the pharmaceutical industry towards new discoveries that could guarantee a healthier and longer life for everyone.